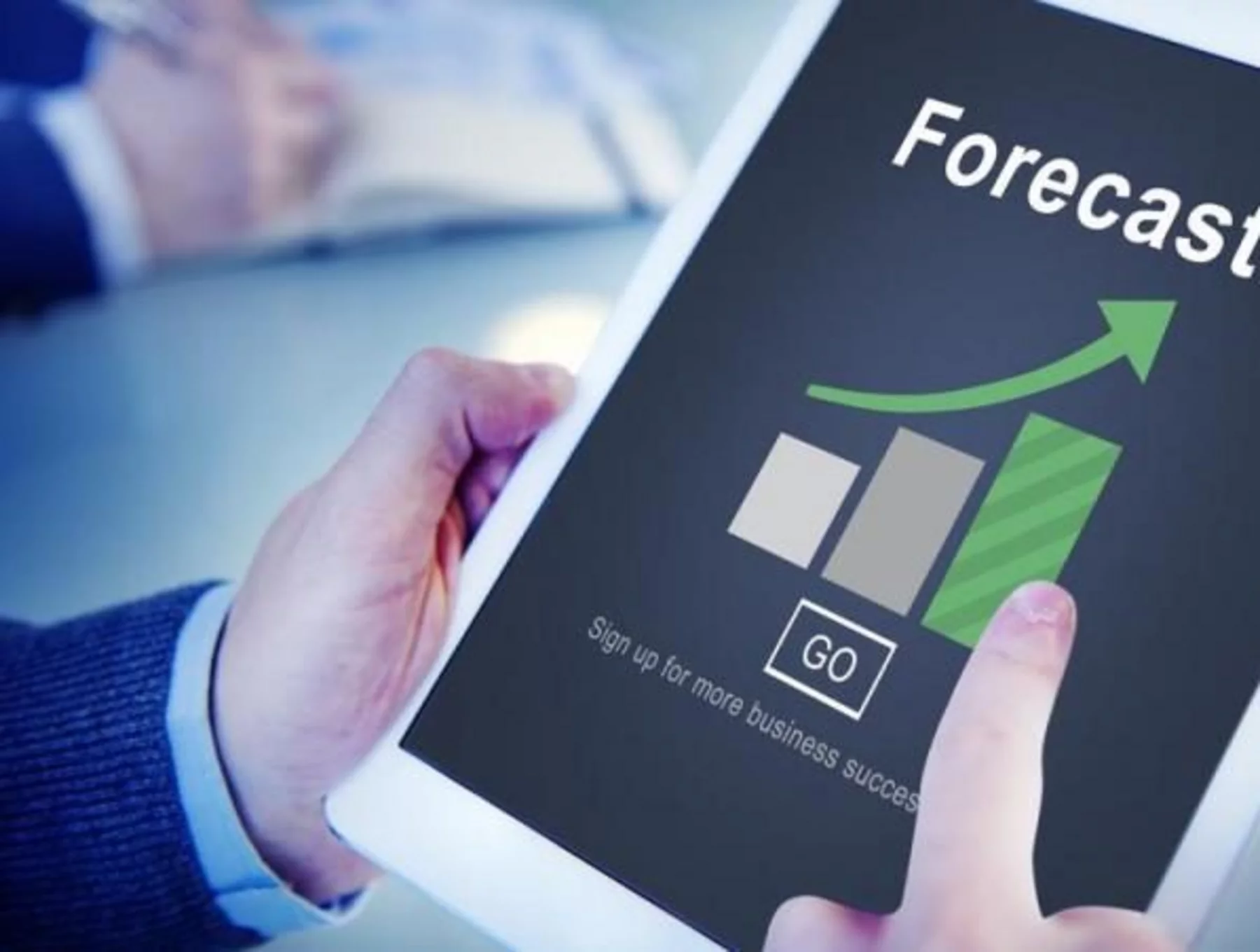
Supply chain forecasting plays a critical role in the success of modern businesses. As markets become increasingly dynamic and customer demands evolve, accurate predictions of future demand have become imperative for companies to stay competitive and efficient. Supply chain forecasting involves analyzing historical data, market trends, and other relevant factors to anticipate the quantity of goods or services required in the future. This vital process enables businesses to optimize inventory levels, production schedules, and distribution plans, ensuring products are available when and where customers need them. In this essay, we will explore five key reasons why supply chain forecasting is of paramount importance, impacting inventory management, cost efficiency, customer satisfaction, production planning, and risk mitigation.
What is supply chain forecasting?
Supply chain forecasting is the process of predicting future demand for products or services in a supply chain network. It involves using historical data, statistical models, and other relevant information to make informed estimates about the quantity of goods or services that will be needed at different points in the supply chain. The primary goal of supply chain forecasting is to optimize inventory levels, production schedules, and distribution plans to meet customer demands efficiently while minimizing costs and waste.
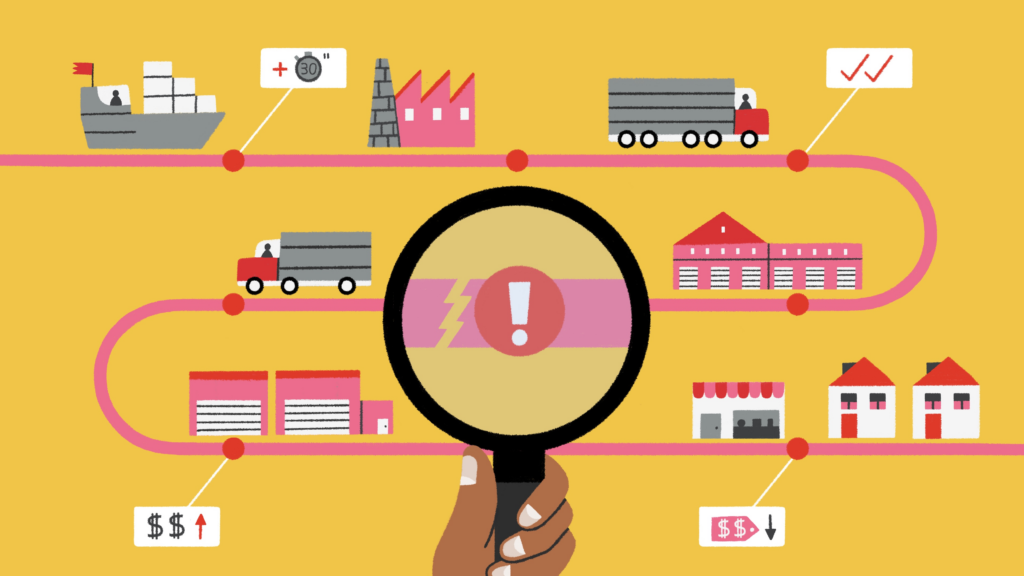
There are various forecasting methods, ranging from simple time series models to more advanced statistical techniques and machine learning algorithms. The choice of method depends on the availability of data, the nature of the products or services, and the level of accuracy required for the specific supply chain context. Continuous monitoring, feedback, and improvement are essential aspects of effective supply chain forecasting, as it helps businesses adapt to dynamic market conditions and maintain a competitive advantage.
Why is supply chain forecasting important?
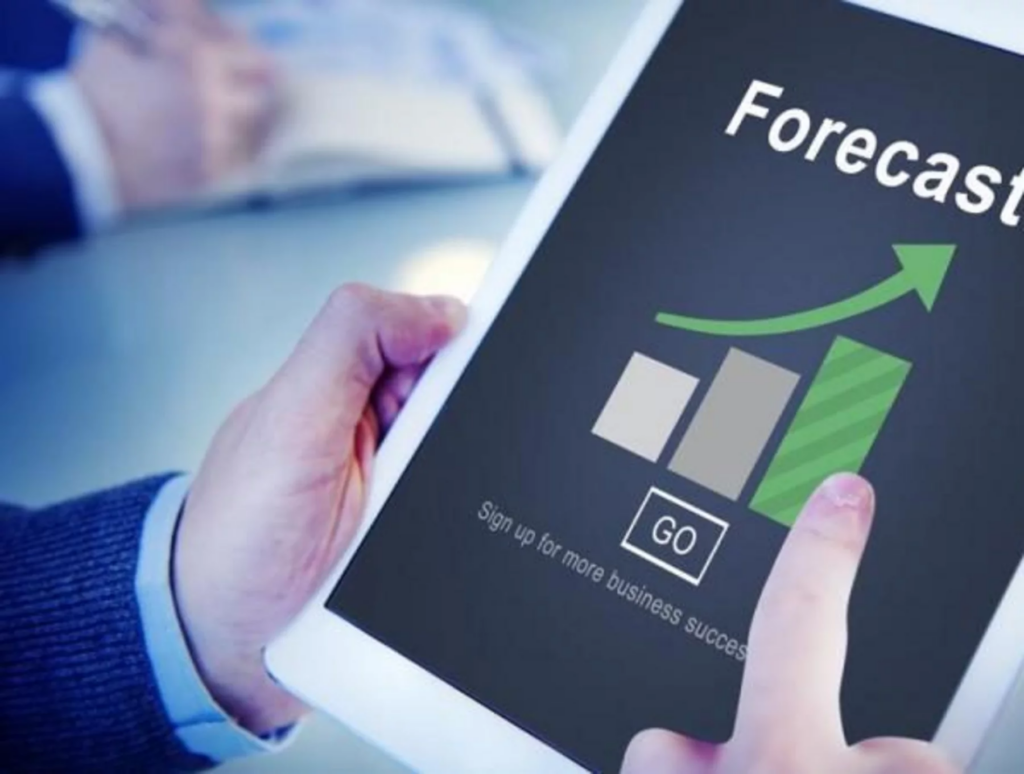
Supply chain forecasting is important for several reasons that significantly impact the efficiency, profitability, and overall success of businesses. Here are five key reasons why supply chain forecasting is crucial:
Inventory Optimization
Accurate forecasting allows businesses to maintain optimal inventory levels. By predicting future demand, companies can align their inventory levels with customer needs, avoiding excessive stockpiling that ties up capital or stockouts that lead to lost sales and dissatisfied customers. Inventory optimization helps reduce holding costs and wastage while ensuring products are available when and where customers want them.
Cost Management
Effective supply chain forecasting contributes to better cost management. By aligning production and distribution activities with anticipated demand, companies can optimize their resources, reduce inefficiencies, and minimize unnecessary expenses. This leads to cost savings across the entire supply chain, ultimately increasing profitability.
Customer Satisfaction
Satisfying customer demands is a top priority for businesses. Accurate forecasting ensures that products are available when customers want them, leading to improved service levels and higher customer satisfaction. Happy customers are more likely to become repeat buyers and brand advocates, contributing to long-term success.
Production and Capacity Planning
Forecasting helps manufacturers plan their production schedules and allocate resources efficiently. It enables companies to ramp up or scale back production capacity based on expected demand, reducing lead times and optimizing production processes. This results in improved production efficiency and reduced idle capacity.
Risk Management
Supply chain forecasting plays a crucial role in identifying potential risks and uncertainties. By predicting demand and market trends, companies can proactively plan for supply chain disruptions, changes in consumer preferences, or economic shifts. Having contingency plans in place allows businesses to mitigate risks and respond effectively to unforeseen events.
In summary, supply chain forecasting is essential for achieving operational excellence and maintaining a competitive edge in the market. It helps businesses make informed decisions, optimize their supply chain processes, meet customer demands efficiently, and adapt to changing market conditions. By integrating accurate forecasting practices into their supply chain management, companies can drive growth, reduce costs, and enhance customer satisfaction.
What is the best method of supply chain forecasting?
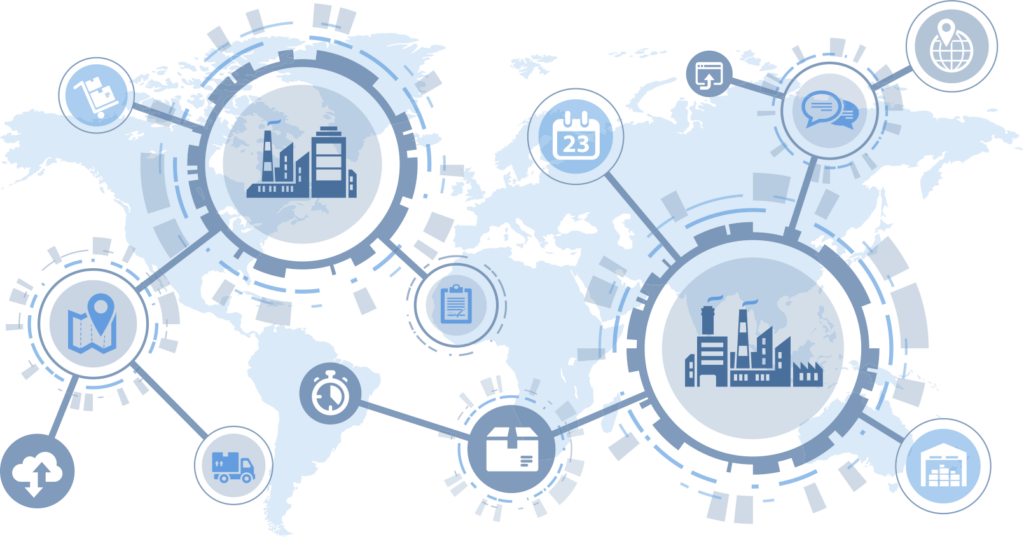
The “best” method of supply chain forecasting can vary depending on the specific industry, products or services involved, available data, and the level of accuracy required. There is no one-size-fits-all approach, and different forecasting methods may be more appropriate in different situations.
Here are some common forecasting methods used in supply chain management:
Time Series Forecasting
Time series forecasting involves analyzing historical data to identify patterns and trends over time. Methods like Moving Average, Exponential Smoothing, and ARIMA (AutoRegressive Integrated Moving Average) are commonly used in time series forecasting. It works well when there is a consistent and stable historical pattern to predict future demand.
Regression Analysis
Regression analysis is used when there is a causal relationship between the variable being forecasted (e.g., demand) and other related variables (e.g., price, marketing spend, etc.). It helps quantify the impact of these factors on demand and can be useful when there are clear cause-and-effect relationships.
Machine Learning Techniques
Machine learning algorithms, such as neural networks, decision trees, and random forests, can be powerful tools for supply chain forecasting. These techniques can handle large datasets, non-linear relationships, and complex patterns in data. They often outperform traditional statistical methods when there are significant uncertainties and nonlinearities in the data.
Judgmental Forecasting
In situations where historical data is limited or unreliable, judgmental forecasting involves gathering expert opinions and insights from key stakeholders. Delphi method and market surveys are examples of judgmental forecasting techniques.
Collaborative Forecasting
Collaborative forecasting involves leveraging data and insights from multiple entities in the supply chain, including suppliers, manufacturers, distributors, and retailers. This approach can enhance the accuracy of forecasts by considering various perspectives and capturing more market intelligence.
Hybrid Approaches
Often, combining multiple forecasting methods can yield more accurate results. Hybrid approaches use a combination of different techniques to leverage their respective strengths and mitigate weaknesses.
The choice of the best method will depend on factors such as the availability of historical data, the level of complexity in demand patterns, the resources and expertise available, and the desired level of accuracy. It’s essential to continually evaluate and improve the forecasting process and adapt to changing market conditions and business requirements.
Moreover, forecasting should be viewed as an iterative process, where regular monitoring and feedback are used to improve the accuracy of future predictions. Companies may use a combination of short-term and long-term forecasting methods to handle different planning horizons effectively. By continually refining and adjusting their forecasting techniques, organizations can optimize their supply chains and make more informed decisions.
How does supply chain forecasting work?
Supply chain forecasting works through a systematic process that involves data analysis, statistical modeling, and collaboration to predict future demand for products or services.
Here are five key steps that illustrate how supply chain forecasting works:
Data Collection and Preprocessing
The first step is to gather historical data on product demand, sales, and other relevant factors from different sources within the supply chain. This data may include sales records, customer orders, inventory levels, marketing promotions, economic indicators, and seasonal patterns. Once collected, the data is cleaned, organized, and processed to ensure accuracy and consistency.
Time Series Analysis
Time series analysis is a fundamental technique used in supply chain forecasting. This involves examining historical data over time to identify patterns, trends, and seasonality. Various statistical methods like Moving Average, Exponential Smoothing, or ARIMA are employed to detect recurring patterns in the data, which can serve as a basis for predicting future demand.
Selection of Forecasting Method
Based on the insights gained from time series analysis and the nature of the data, an appropriate forecasting method is chosen. Different techniques may be applied, such as simple forecasting models for stable demand patterns or more sophisticated machine learning algorithms for complex and nonlinear trends.
Collaborative Forecasting and Input Integration
In many supply chains, collaboration is essential for accurate forecasting. Businesses may collaborate with suppliers, manufacturers, distributors, and retailers to share information, insights, and market intelligence. This collaborative approach helps integrate various factors that could influence demand, such as promotions, product launches, or changes in consumer behavior.
Model Validation and Adjustment
After building the forecasting model, it is essential to validate its accuracy using historical data that was not used during model development (known as validation data). The model’s performance is evaluated, and if necessary, adjustments are made to improve its accuracy. Regular monitoring and updates are required to ensure the forecasting model remains relevant as market conditions change.
By following these steps, supply chain forecasting helps businesses make informed decisions about inventory management, production planning, distribution, and resource allocation. It minimizes inventory carrying costs, reduces stockouts, enhances customer satisfaction, and enables businesses to align their operations with anticipated demand. Successful supply chain forecasting contributes to improved efficiency, profitability, and overall performance in a competitive marketplace.